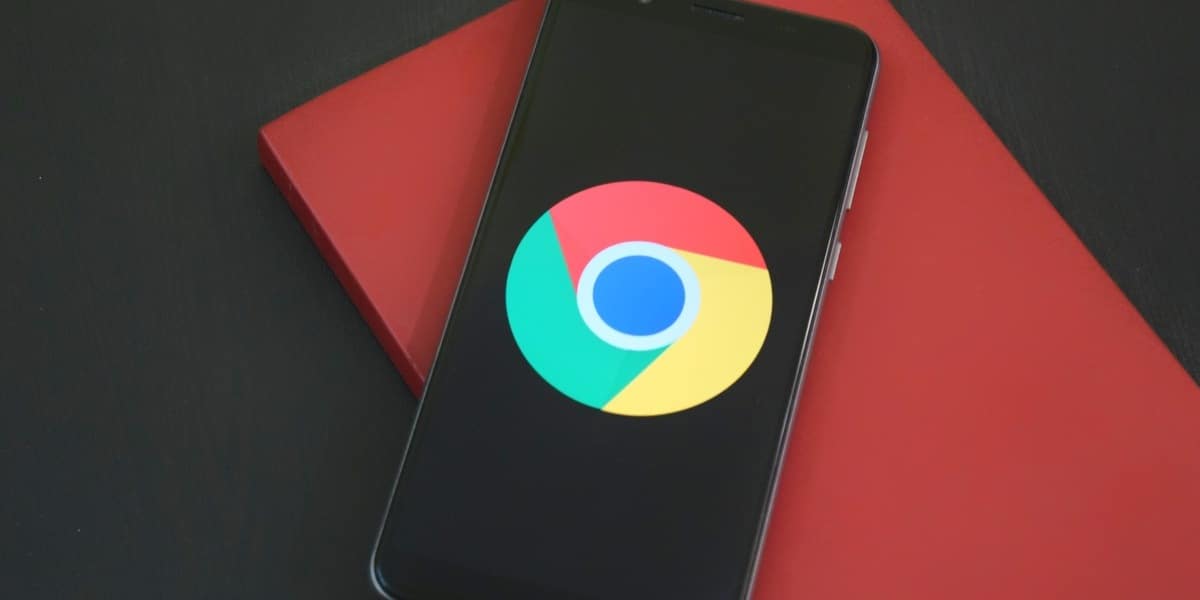
How will the May 2022 google core update affect your site?
20 June 2022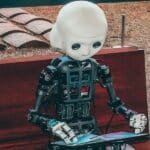
AI in image generators
15 September 2022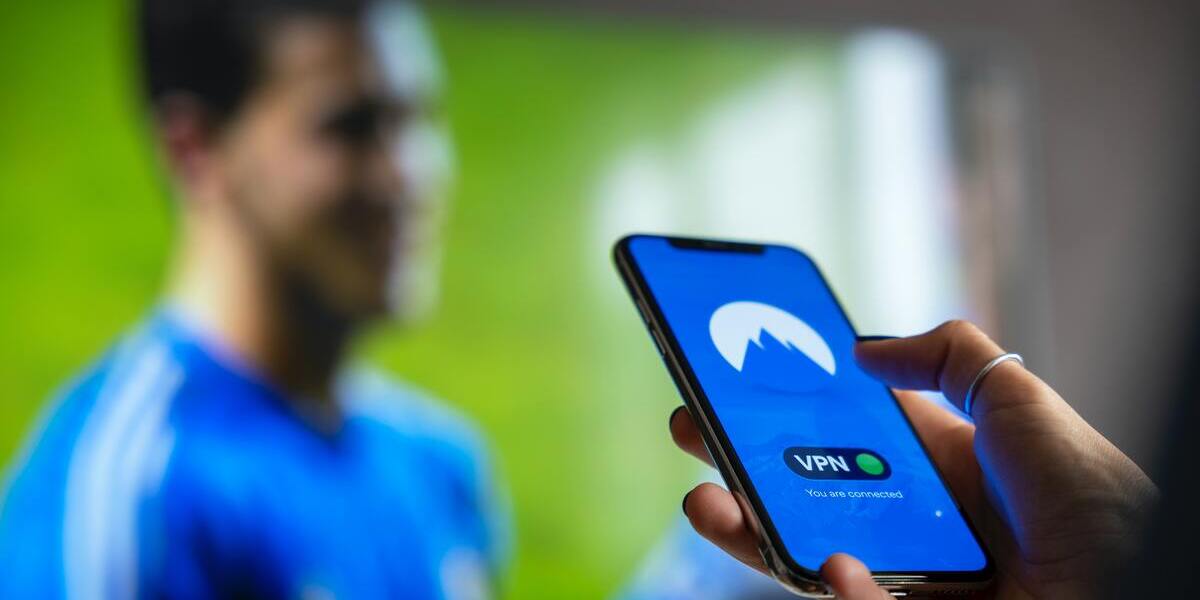
The era of big data has brought an unprecedented impact on the development of the sports industry. Services closely related to it, big data services, can effectively assist athletes in their daily training and game strategies, becoming an indispensable means of winning competitions. Advanced big data technology has brought changes to the field of sports. The proliferation of sports data has created new opportunities and challenges in the field of big sports data. Sports big data is a product of the development of the Internet and sports.
Big Data management in sport
Managing big sports data is mainly about data management techniques, tools and platforms to deal with it. However, managing large data sets is a complex process. The main goal of big data in sports is to extract the potential value of big sports data and to improve the quality and availability of data for decision-making.
Data collection in sports
An especially important characteristic of sports big data is their diversity. Data sources are not only incredibly broad, but also the types of data are highly complex. The development of the Internet of Things, the internet, and the sports industry has enriched the amount of sports data. As network data is diverse, complex in composition, and has varying methods of utilization, collecting large datasets regarding online sports is also very difficult. Big data in online sports is usually gathered by indexing robots.
The crawler’s overall data collection process includes the following six aspects:
- website analysis,
- extracting links,
- link filtering,
- content extraction,
- URL queuing
- data search
Currently, applications for managing large datasets are popular but face challenges and problems related to security. Collecting large sports datasets is a key task in data processing. Moreover, secure collection of large sports datasets is an essential step for all kinds of data applications that can provide results of large data analysis. Because suspicious data sources enable data collection to investigate various malicious attacks, secure methodology for collecting large sports datasets is crucial for different data applications. Effective frameworks have been proposed to overcome these issues, based on blockchain and reinforcement learning.
Big data labeling in sports
Once enough sports data has been collected, the next step in processing is to mark individual examples. Typically, data collection is done along with data labeling. When extracting information from the web and constructing the knowledge base, each piece of information is assumed to be correct, and thus is marked as true by default.
Data labels fall into three categories:
- Existing labels. These existing labels can be used to learn from them to predict other labels.
- Crowd driven. Recently, many crowdsourcing techniques can be used to help gamers make their labeling more effective.
- Poor labels. While it is desirable to generate correct labels all the time, this implementation process can be very expensive. Weak label is an alternative method that is used in many applications as labeled data.
Improvement of existing data
Machine learning technology can be used to handle noisy data and uncorrected labels. There are many publications on improving data quality. The cleaning system constructs a probabilistic model that utilizes quality rules, value relationships, and reference data to capture how data was generated. Furthermore, other data cleaning tools have been developed to convert raw data into a better form for further analysis. To obtain high-quality labels with data, a good solution is to improve the quality of existing labels. They investigate the improvement (or lack thereof) of data quality through repeated labeling and focus on improving training labels for supervised induction.
Big Data analysis methods in sport
Big data analytics refers to a technique that allows you to quickly extract valuable insights from all kinds of data. The big data analytics technique can use various algorithms to statistically calculate big data and extract important analytics data to meet real needs.
Below we present the methods of Big Data analysis in sport:
- Statistical analysis
Based on the statistical theory, a technique of statistical analysis was proposed, which belongs to the branch of applied mathematics. Statistical analysis can provide conclusions for large data sets. In sports industry research, the technique of statistical analysis is typically used to process sports datasets. By analyzing some statistical characteristics of sports datasets, such as mean, variance, entropy, and maximum/minimum value, an athlete’s movement pattern can be investigated, and based on this statistical analysis, coaches can develop an effective training plan
- Sports social network analysis
Analyzing sports social networks can reveal relationship patterns in team sports. A social network analysis technique is used to identify some of the variables related to Twitter influence. A network approach within positioning-based variables is used to identify individual players’ contributions to the outcome of a team’s behavior during a simulated match.
- Big data analytics services platform for sports
It is a big data analytics platform that stores and analyzes massive data to form a big data mining system
Recently, to promote the development of big data analytics in the intelligent sports area, more and more researchers are paying attention to distributed intelligent sensing technology. Based on the sports big data platform, the game relationship between profit and consumption intention in the sports hall is analyzed.
How sports analytics is changing the game
Data analytics has changed the game and is essential to helping team managers, coaches and players ensure they are prepared to win. Since preparation is the key to victory, professional teams take sports analytics seriously and collect as much data as possible to ensure a competitive advantage.
Some of the most important metrics that teams analyze before a match include:
- Opposing player stats, such as typical plays or setups and scoring types.
- Recent wins and losses, and how each player’s performance contributed to those games.
- The weather conditions on the day of the match and the experience of the players in these conditions.
- Game stats, including the number of games they need to win to make the playoffs or break previous records.
Professional sports teams are working hard to collect the right data to prepare for the games. There are many ways that players, teams and fans use statistics and data to improve their position.
Player analysis
To improve performance, players track their own stats and analyze how they performed in previous games. Nutrition, training hours, and in-game scores create different types of stats, such as an athlete’s running speed, the weight they lift, or the amount of protein they ate in a day.
By tracking this data and comparing it to how they felt on game day or how they performed, players can make adjustments to their training routines or diets to improve their game. When all players focus on analyzing their results and pinpointing areas for improvement, this analysis and the resulting changes can help prevent situations where organizations become the most disappointing teams in the league.
Team analysis
Each player must focus on individual performance, but playing together as a team is also crucial to ensure victory. When team members implement data analytics together, they can analyze their results together.
Coaches can experiment with player combinations to see if you get better stats with different lineups on the pitch. Using data analytics, team managers can develop machine learning techniques to identify winning combinations of players and effective strategies.
Fan analysis
Sport is a business and the more involved fans are, the more profit organizations make. By learning about online data analytics, sports management teams can learn how and when fans are likely to attend events or purchase merchandise.
Executives analyze social media, attendance and merchandise sales to better understand what consumers expect from the game. This allows them to define what is important to the fans. Management can then provide facilities to keep fans happy and coming back.
With fans in mind, management can develop marketing strategies and advertising campaigns aimed at these consumers. The data helps them easily identify fans who are likely to engage with the team so as not to spend money advertising consumers who are not interested in their sport.
Gambling in sports
When players can analyze a team’s past performance with accurate stats, it’s much easier to predict when and where a team will succeed in the future.
Sports fans, without having to blindly choose which team or player will perform well, are more likely to engage in gambling. Statistics allows them to develop a data-driven prediction method, making bettors feel more confident betting on specific teams or players.
Athlete safety and data analysis
Athlete injuries can wreak havoc on a season or team performance. When star players drop out due to an avoidable injury, it’s frustrating for coaches and can have a negative impact on a player’s career. While some injuries are unavoidable, data analysis helps players and medical staff understand when and how often injuries occur.
With the information, players can identify weaknesses in their form so they can be more careful while playing. Sports medicine professionals can also analyze how they have treated injuries to better understand their success rate. They may change treatment plans for certain injuries or players to try to speed up healing.
Scouting
Talent scouts typically observed potential players practicing or playing in games. However, data visualizations and analyses that include statistics about past performances are also an important part of the recognition process. Professional scouts cannot always physically visit every promising college player, so they rely on stats to determine and prioritize who to visit and watch.
Data is an important part of the sports industry for players, coaches, managers, sports medicine professionals and fans. Not only can data analytics help teams win games, these stats can also help improve player performance, prevent injuries and encourage fans to attend games.