Artificial Intelligence (AI, Machine Learning)
Adaptive self-learning solutions based on large amounts of data and machine learning algorithms will throw out from the market hard-coded, expensive, first-principles-based, inflexible solutions in the next 6 years. AI provides solutions to problems in a non-linear way, much closer to production reality, and also significantly reduces the margin of human error.
AI: A GAME CHANGER FOR INNOVATION
What benefits does AI provide?
Faster decision making
Advanced algorithms and a better understanding of the context facilitate building more precise automations in business and intelligent technological processes. Scale your business faster!
Productivity gains
The AI engine built and implemented by us increased the efficiency of contamination detection by over 60% during production and accelerated the operation of the production line by 2x.
New capabilities
Companies that have been collecting data for a long time will gain an incredible advantage over the competition. AI increases capabilities and efficiency in many technological fields.
Reduction of human error
OpenAI surprises with its capabilities, and the development of AI will actually threaten many professions on the labor market, precisely because of its effectiveness and stability in operation.
Solutions based on machine and deep learning are used in robotics, quality control, data aggregation or object recognition. AI is also very useful in e‑commerce.
EXPLORE POSSIBILITIES
What can machine learning be used for?
Predictive modelling
Predictive modeling is a process that uses data mining and probability to predict outcomes. Each model consists of multiple predictors, which are variables that can affect future outcomes. After collecting data for the relevant predictors, a statistical model was formulated.
- Customer life cycle value forecast
- Anomaly/fraud detection
- Dynamic pricing
- Demand forecasting
Text processing
Language processing broadly refers to the study and development of computer systems that can interpret speech and text as humans naturally speak and write it. One text can be converted to many other outputs and processed on the fly.
- Analytics of social media profiles
- Smart chatbots
- Sentiment analysis
- Document categorization
Computer vision
Computer vision is about automatically retrieving, analyzing, and understanding useful information from a single image or sequence of images. It involves developing a theoretical and algorithmic foundation to achieve automatic visual understanding.
- Object detection
- Image and video processing
- Content indexing
- Scene segmentation
TECH STACK
What technologies do we use?
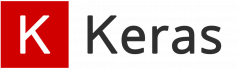
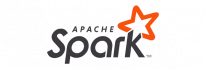
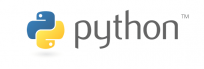
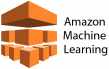
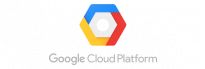


How smart applications work
Research on machine learning, artificial intelligence and intelligent applications has accompanied the development of computerization since its inception, but at the beginning the possibilities of their effective use have been limited by the computing power of computers. The development of new generation processors, computing devices and GPUs has made practical the application of machine learning assumptions on a larger scale.
Solutions based on machine learning allow for much more efficient data processing and automatic response according to established assumptions.
An important element of solutions based on machine learning is the possibility of automatic modification of algorithms by the program. The system checks the quality of its own output data, comparing it to the assumed values, and then automatically modifies its algorithms to improve the quality of these results, so it does not require continuous intervention and manual change of parameters.
The machine is still no substitute for all the tasks of a real analyst, although appropriate, well-prepared solutions based on machine learning will relieve it very much at work. The machine will replace it in viewing statistics, sheets and charts, but it should still be programmed for this purpose, periodically check the effects, provide new data, and modify the algorithms of neural networks.
Creating and optimizing models
Deep machine learning is based on computational operations that take place in neural networks, consisting of a set of hierarchically ordered, non-linear functions. The structure of the neural network is modeled to some extent on the connections between nerve cells of the living body’s brain, and the data in this model are analyzed and processed non-linearly, in multidimensional matrices and using fuzzy logic methods, i.e. not zero-one, but taking intermediate states into account, gradual, contradictory or ambiguous.
The effectiveness of machine learning depends on the quality of algorithms and input data, and finding the right patterns is fraught with certainty.
In summary, the machine sorts huge amounts of input data and selects those that it considers valuable. It uses them in the way given by a neural network programmer, and at the same time modifies its own algorithm to a certain extent in order to fulfill the given assumptions.
Tensorflow
Tensorflow is an advanced, flexible and convenient tool for creating, processing and analyzing multidimensional and multi-layer data matrix in deep learning. Built-in diagnostic tool Tensorboard enables a convenient analysis of data flow in the form of expandable, scalable charts.
Tensorflow is a product of Google, which originally developed this system for internal needs, and in 2015 released it under an open license for free use. Currently, Tensorflow libraries and tools are available on all popular devices and operating systems.
Examples of applications
In practice, solutions based on machine and deep learning in robotics, automation, in production processes, quality control, data aggregation, for identifying people and objects, voice recognition are used, wherever there is a need to automate processes or tasks.
Modern automated cleaning robots use machine learning techniques for indoor orientation or for dirt detection algorithms. Devices responding to voice commands are configured to understand these commands as accurately as possible and respond appropriately to them, for example, including emotional tone of the voice, changes in key tones or even simple hoarseness.
Anti-spam filters that use machine learning are more effective than traditional ones and better capture unwanted content, and also modify their own spam detection algorithms, thanks to which they automatically adapt themselves to changing spam techniques.
Marketing content is personalized automatically based on data about shopping habits and customer interests.
Search algorithms use machine learning when sorting and matching content. The use of ML methods for the analysis of Internet traffic enabled the operation of large data sets and translated into an increase in the accuracy of results, whereas in predictive analytics, the use of machine learning increased the accuracy of predictions.
AI is also implemented in areas outside the strict IT industry. In medicine, e.g. in epidemiology, in the analysis of the spread and prevention of diseases.
In the end: progressing work on autonomous vehicles is another thing possible thanks to artificial intelligence and machine counting. Such a car has to analyze very quickly the huge amount of information obtained from cameras and car sensors, and react immediately in situations where human life is at stake.
TESTIMONIALS
Over 400 projects for 140 clients
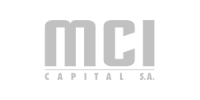
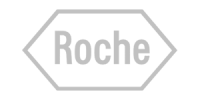
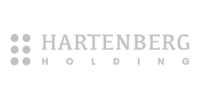
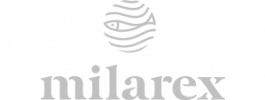
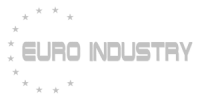
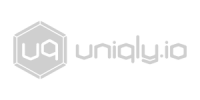
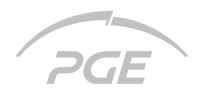
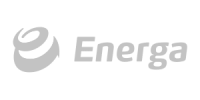
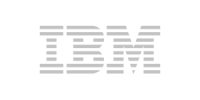
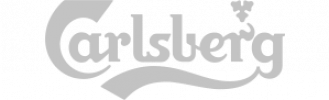
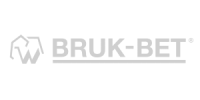
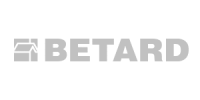

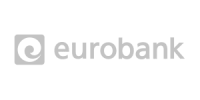
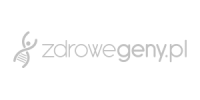
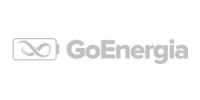
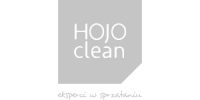
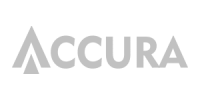
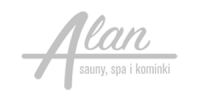
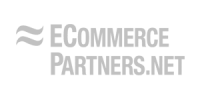
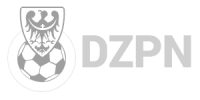
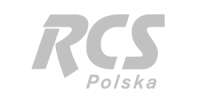
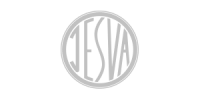
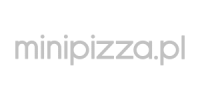

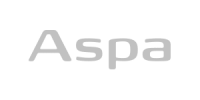
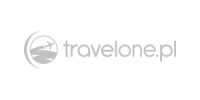
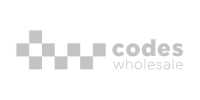
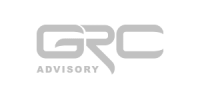
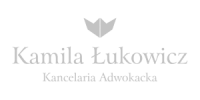
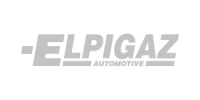
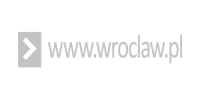
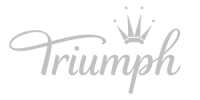
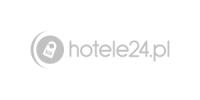

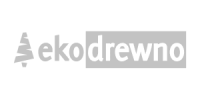
want to
talk with us?
Leave your details and one of our experts will contact you.