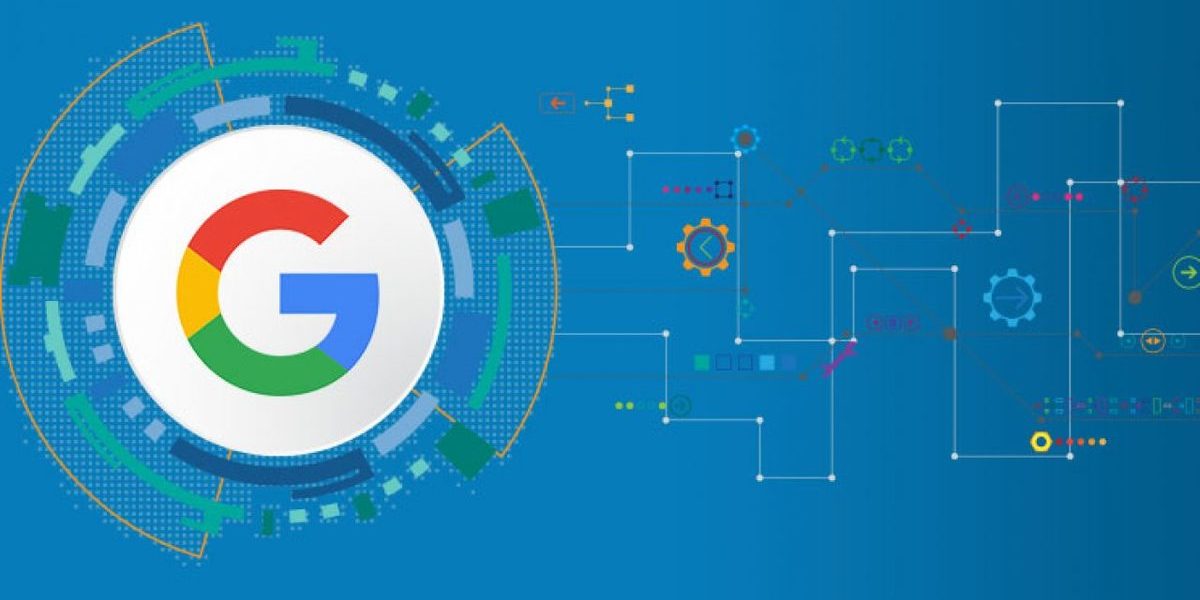
Google’s spam update
13 August 2021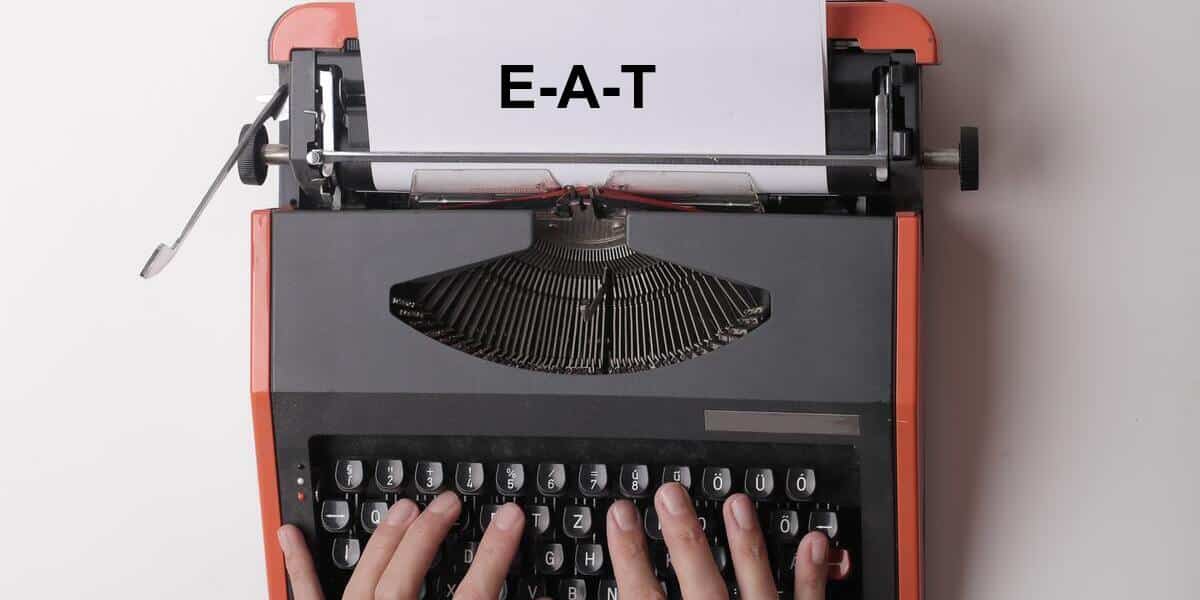
What is E-A-T and what does it mean for Google?
11 October 2021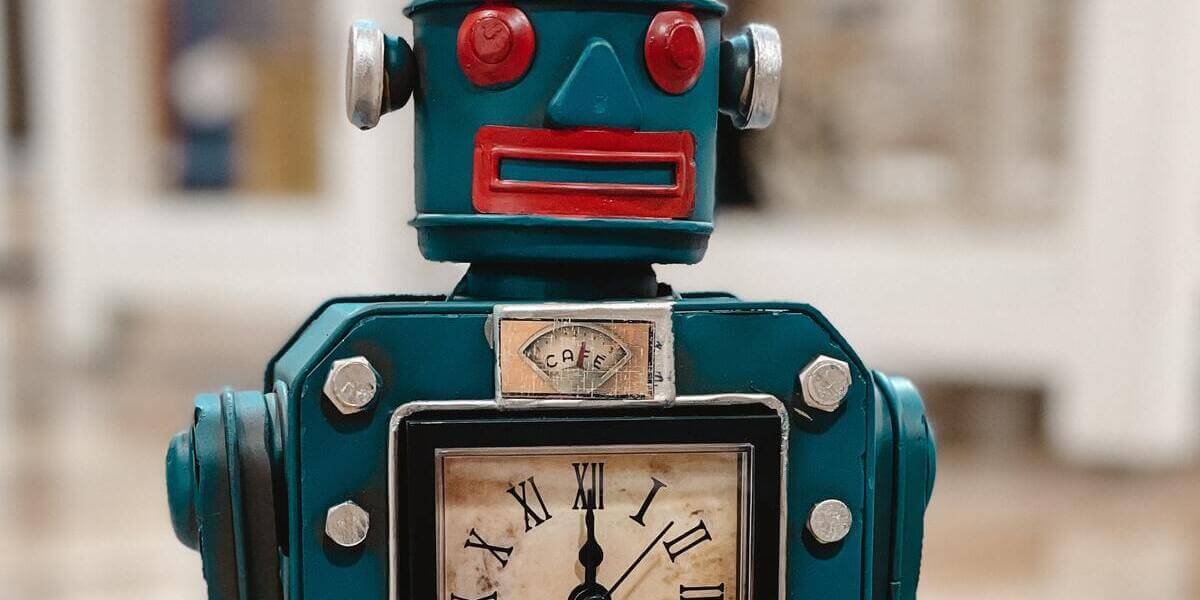
Natural language processing (NLP) is one of the fastest-growing sectors in artificial intelligence(AI) and machine learning (ML). The global NLP market is expected to reach $40 billion by 2026.
NLP has come a long way since its beginning. As the amount of unstructured data increases, NLP technology continues to evolve to better understand the nuance, context, and ambiguity of human language. Today, artificial intelligence can not only speak, write, listen and understand human language but also extract meaning from natural language to make informed decisions all through natural-language processing. NLP is probably one of the most exciting fields in artificial intelligence and has already given rise to technologies such as voice assistants, chatbots, translators, and many other tools that we use every day.
Natural language processing applications
Natural Language Understanding (NLU)
NLU goes beyond simply processing words as data – it is designed for computers to understand what a human is saying. Instead of turning words into codes, NLU takes human syntax, sentences, and structure and makes decisions based on the way the person speaks.
Sentiment analysis
Sentiment analysis takes into account not only what was said but also the emotions behind it. It is especially useful for B2C businesses, such as seeing social media discussions about a product.
Machine translation
Machine translation is the process of using a machine to convert text from one language to another, for example, using a translation application. Many apps translate word for word, substituting at a very standard level, and the structure of the context or sentence can get lost in translation.
Chatbots
If you’ve been browsing or shopping online, you’ve probably interacted with chatbots. Chatbots are customer service representatives using artificial intelligence or algorithms that use natural language processing to be able to respond to your request. Chatbots use NLP to not only answer your questions but to provide accurate, automated answers in real-time.
Email assistant
Autocomplete, spell check and autocorrect are examples of NLP-enabled features that help you compose emails. Natural language processing is also used by email spam filters to help determine whether an email is a spam or worth keeping.
Semantic parsing
Just as humans have to subconsciously analyze words to understand their meaning, machines do the same. Semantic parsing is the ability to transform natural language data into something that a machine can understand on its own terms.
Applications of NLP in Data Science
NLP has many applications in the data science industry, which then translates to other fields, especially in terms of business value.
Speech recognition
Behind speech recognition is NLP. By analyzing speech patterns, meaning, relationships, and word classification, the algorithm is able to assemble a sentence into a complete sentence. Using DeepLearning, you can also teach the machine to recognize your accentor speech disorder to be more accurate. Additionally, technology allows people with disabilities to communicate with machines much more easily.
Market analysis
NLP enables companies to identify current trends by analyzing large amounts of available data. Using the topic classification, the machine can find out which categories are the most common. For example, social media analytics can provide information about your industry, product, or brand straight from the consumers’ point of view, improving your business intelligence.
Prediction of diseases
NLP is widely used in healthcare as a tool to predict possible diseases. NLP algorithms can provide clinicians with information about progressive diseases such as depression or schizophrenia by interpreting speech patterns. Nevertheless, psychiatry is not the only field of medicine where NLP is applied. Medical records are a huge source of information, and practitioners use NLP to detect disease, better understand patients, facilitate care delivery and cut costs.
Search results
Using NLP, search engines can determine the intent of any query. Google uses this technology to give you the best possible results. With the introduction of BERT in 2019. Google has greatly improved intent detection and context. This is particularly useful for voice search, as queries typed in this way tend to be much more conversational and natural. Google turned on BERTmainly because as many as 15% of the queries entered daily had never been used before. This influence has largely shifted the search intent behind them, making the optimization process and keyword research different.
Trends in 2021
This exponential growth of NLPhas also led to new trends and innovations in the industry. Let’s talk about the NLP trends to watch out for in 2021.
Social media sentiment analysis
In social media, a large amount of data is generated at any given time. It also raises the specific problem of making sense of all the generated information, which can not be done manually.
NLP has proven to be an important tool in this regard. Used as a sentiment analysis tool, NLP is helping more and more companies quickly understand how customers think about their brand by analyzing emotions, language and understanding the urgency of the conversation.
However, NLP still suffers from a linking deficiency, that is, an inability to distinguish between different meanings of a word. This includes the contextual meaning of words and sentences and identifying sarcasm or ironic statements. Another challenge facing NLP today is the analysis of statements with multiple meanings, often contradictory.
While it cannot be said with absolute certainty that these problems can be solved in the future close as 2021, there is room for significant improvement in NLP techniques in this area to better analyze emotions and sentiment in social media.
Multilingual NLP
Most advances in NLP have so far focused on English. However, large technology companies such as Google and Facebook are now introducing trained multilingual models whose performance is comparable to monolingual models.
With such innovations and recent advances in language-independent sentence embedding and learning from scratch, we can expect more innovations in the multilingual NLP model space soon.
Transfer Learning
Transfer learning refers to a machine learning (ML) technique in which a model is trained for the main task and then reused for another similar task. So instead of developing and training a new model from scratch, you can simply retrofit an existing model.
This helps save time and resources. As cost reduction will be one of the top priorities for companies moving forward, transfer learning will become a major NLP trend in 2021.
Collaboration between supervised and unsupervised learning
Supervised Learning is a machine learning process that involves mapping input to output based on sample input-output pairs. On the other hand, unsupervised learning does not involve any input-output pairs or learning models. The model works independently to discover potential learning opportunities.
Both supervised and unsupervised learning have their advantages and disadvantages. While supervised learning is more specific and can be helpful in classification problems, its ability to deal with complex tasks is limited. On the other hand, unsupervised learning is advantageous because no labeling data is required, which speeds up the classification task. But on the other hand, it does not allow for mapping or estimation of results.
To bridge this gap between supervised and unsupervised learning, NLP solution providers combine these two learning models. This combination of two models showed to increase the performance of ML models.
Detection of fake news and cyber violence
NLP has become an essential tool to reduce time and human effort in detecting and preventing the spread of fake news and misinformation. With so many fake news stories on Covid-19 this year, we’ve already seen some interesting approaches to automated fake news detection, so we’re sure to see more of them in 2021.
Another way NLP is used is to detect cyber violence. Classifiers are created to detect offensive language or hate speech on social media.
Given the ongoing debate about whether social media content should be regulated, these NLP applications may become even more important.
The future of natural language processing
With the growing volume of information available and increasingly sophisticated, accurate algorithms, NLP is sure to grow in popularity. It changes the way humans and machines interact. The previously mentioned applications of NLP are proof that it is a technology that significantly improves the quality of our lives.
As much as 80% of the information around us is unstructured. For this reason, NLP is one of the largest fields of data science. Cleaning up this data is a major challenge that many professionals face daily. The field of NLP is making steady progress, and we can expect it to affect more and more aspects of our lives.
Natural language processing (NLP) is rapidly evolving, and applications of natural language processing are growing by the minute. With so much data available, it’s important to understand it, monitor it, and in some cases censor it.
In the coming years, NLP will become even more widespread with ready-to-use, pre-trained models. In particular, companies will continue to reap the benefits of NLP, from improving their operations and customer satisfaction to reducing costs and making better decisions.