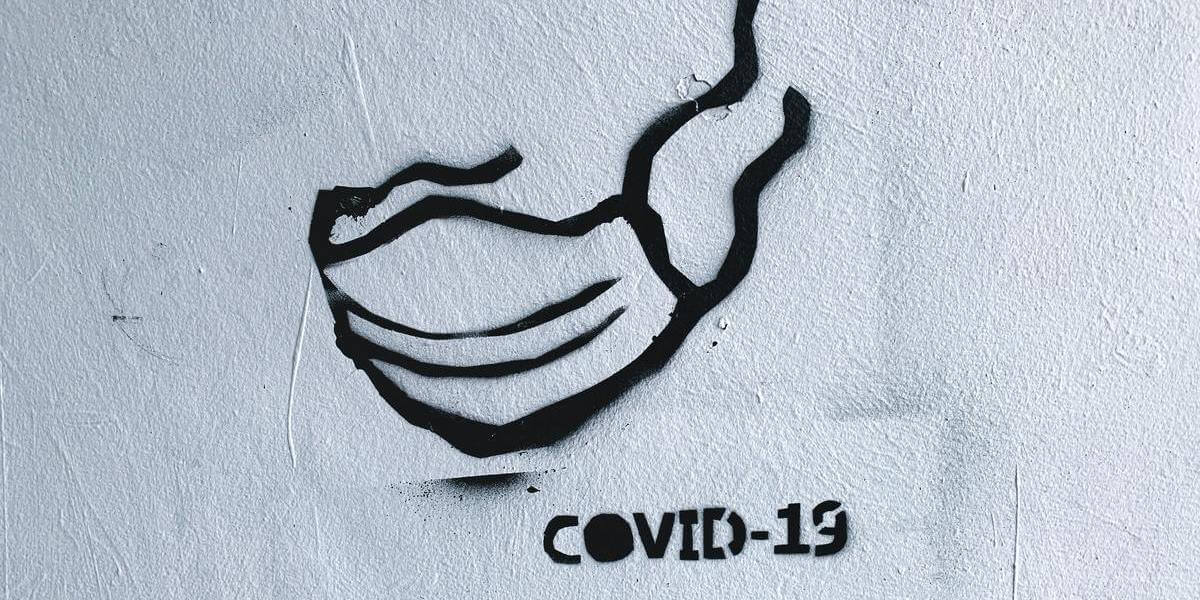
SEO at the coronavirus time. Customize your strategy
15 May 2020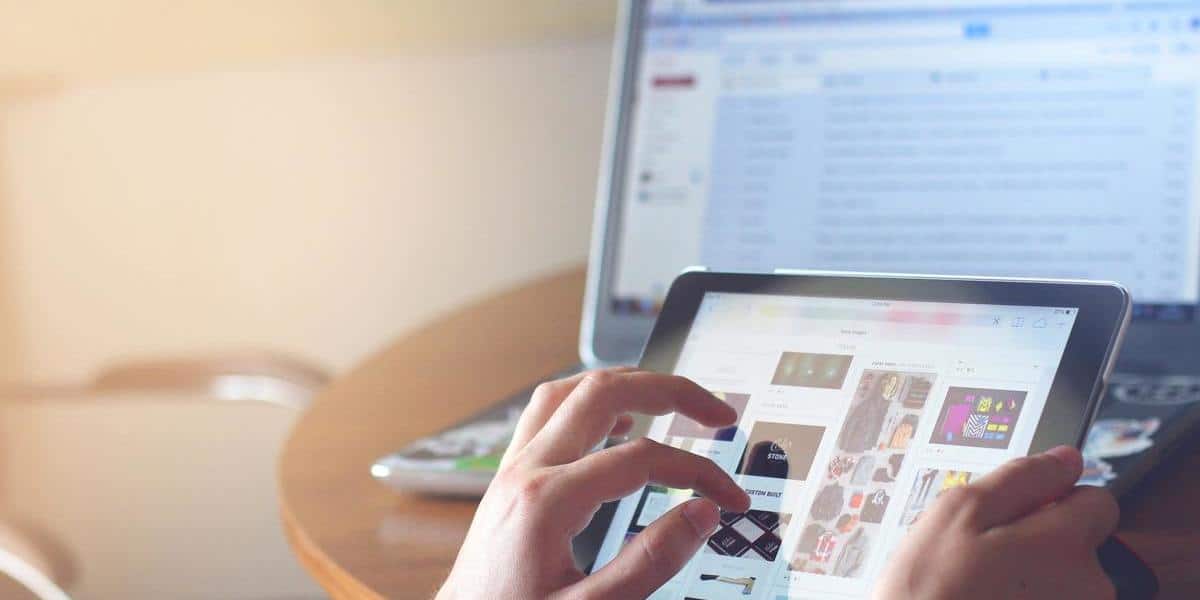
WordPress Ecommerce
29 May 2020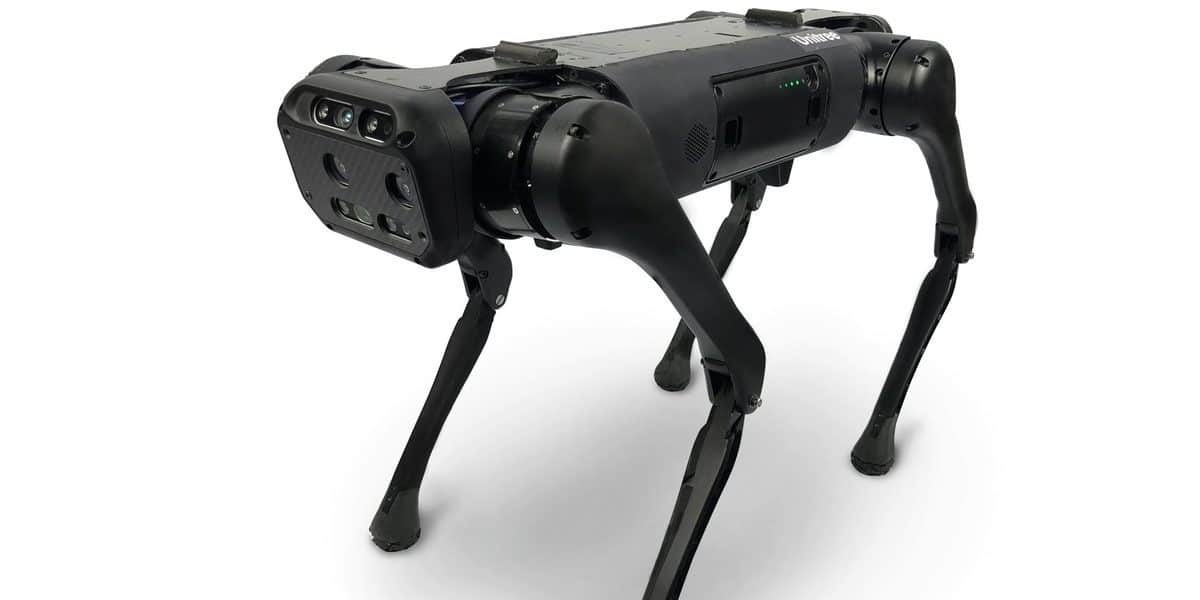
Animal-like robots have the biggest problem with the good coordination of normal walking. At Google, scientists have a secret weapon for teaching robots, and it’s dogs. They collect videos from a public dataset and then feed that data into a simulator to create a digital version of the foursome. Scientists then translate a digital version of a real dog into a digital version of a four-legged robot that has a rectangular body and skinny legs. They then transfer these algorithms to a physical version of Laikago ( the name is no coincidence and comes from the first dog in space – Łajka).
How works Laikago?
A robot works very differently than a normal dog. It has motors instead of muscles and is generally much stiffer. However, through long work, Laikago has learned to move like a real dog. What’s more, its learned gait is faster than the fastest gait provided by the robot’s manufacturer – although, to be fair, it’s still not that stable. The new system could be the first step toward robots that learn to move not through exhaustive coding, but by watching videos of animals running and jumping.
The great advantage of nature is its effectiveness. Every adaptation that could improve the species’ energy use was investigated, and wasteful variants quickly overtook their competitive cousin. We see it in the jumping posture of a cat and the precision of a fish’s jump, even in the rhythm and bounce of our walking. Animals are extremely efficient and adapt to new situations and conditions. Robot designers want their creations to be in a similar way. After all, the basic constraints that nature has been working with for billions of years apply, no matter what the purpose of the robots we create.
The next challenge is that we take what the system has learned in simulation and run it in a physical robot. This is difficult because a simulation is an imperfect and highly simplified version of the real world. Mass and friction are represented as accurately as possible, but not perfectly. The actions of a simulated robot in the digital world do not accurately replicate the movements of a real robot in the lab.
The team behind Laikago has built not one final simulation of the robot, but a range of capabilities that determine the robot’s behavior. For example, they randomized the friction in the simulation and improved the delay between sending a command to the robot and executing the command.
Incidentally, all of these strategies are reasonable for a robot – they don’t want it to move so fast or violently that it injures itself or people. The system has already made the most catastrophic mistakes in the computer simulation, so the robot doesn’t have to make them in the real world. But some of these behaviors result in a better gait than others. Eventually, they acted like dogs, despite the lack of robotic anatomy; the scientists even had the non-existent tail chasing, spinning in circles.
To be clear, this isn’t the first time robots have looked to the animal movement for inspiration. Boston Dynamics’ point robot is obviously modeled after the fluid motions of quadrupeds, and its humanoid Atlas model is modeled after the motions of humans. Using such inspiration, Spot can climb the most difficult terrain thanks to meticulously coded control algorithms.
If we want robots to be useful in an environment like the home, they will have to learn just like we do. It will take a lot of work to create such a library of movements that would be useful for robots with legs.
Navigating the world of people
Unlike most animals, we want robots to be effective not only in the natural environment but also in the human realm. This means that we create robots adapted to a world designed by humans. The clear similarities between robots and living beings in physical design and behavior encourage us to wonder why these machines should be so realistic. We should remember that when we try to build machines that operate in our world of culture and prehistoric survival, we impose the same constraints on them that those worlds impose on us. As we demand more of our machines in the human world, it should come as no surprise that they often start to look more and more like us. Whether we make a conscious choice to copy nature or try to design an efficient machine based on first principles, the results are likely to be the same.
Overall, our system was able to reproduce a fairly diverse corpus of behavior using a four-legged robot. However, due to hardware and algorithmic limitations, we were unable to mimic more dynamic movements such as running and jumping. Learned rules are not as reliable as the best hand-designed controllers. Exploring techniques to further improve the efficiency and robustness of learned rules can be a valuable step towards more complex real-world applications. Extending this framework to learn skills from videos would also be an exciting direction that could greatly increase the number of data robots can learn from.